
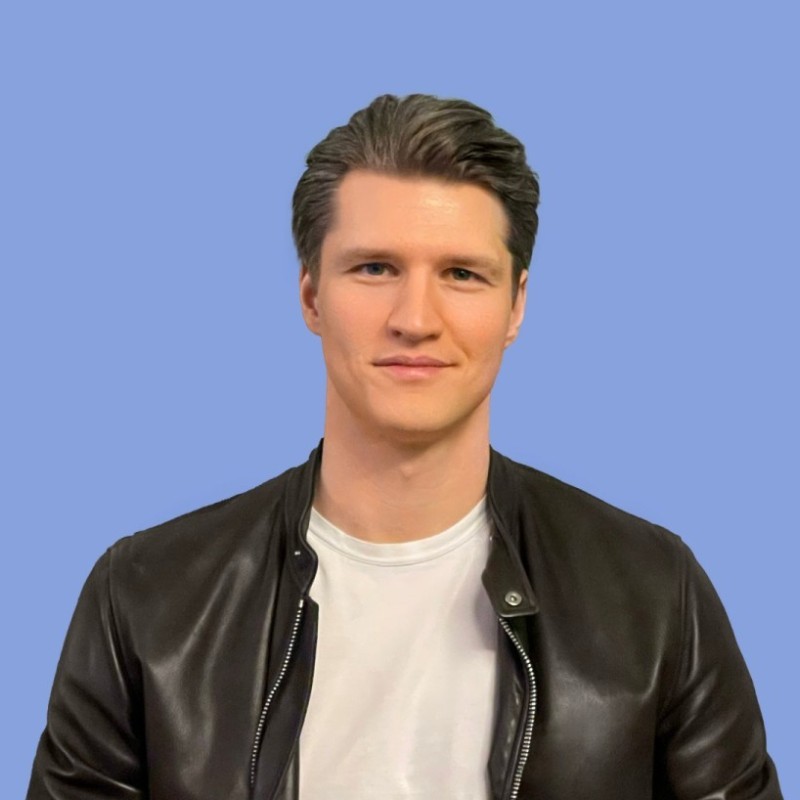
The 5 Levels of AI Autonomy: From Co-Pilots to AI Agents
What is autonomy in AI? AI autonomy refers to an AI system’s ability to operate and make decisions with minimal or no human intervention. In practical terms, it’s the degree of decision-making power and independence an AI has in executing tasks. This ranges from simple rule-following programs to intelligent agents that self-learn and act on their own. Autonomy in AI is significant because it determines how much human oversight is needed – and thus how far businesses can streamline operations. By giving AI more agency, organizations can dramatically increase the number of tasks and workflows that can be automated, driving efficiency and productivity gains. For example, a truly autonomous AI could analyze multiple business systems overnight and decide on necessary actions while employees sleep. This capability to act independently around the clock can enhance decision-making speed, consistency, and scale beyond human limits. It’s no surprise, then, that nearly every industry is investing in AI autonomy. McKinsey reports that almost all companies are investing in AI, yet only about 1% feel they have fully matured and integrated AI into their workflows – highlighting both the enthusiasm for autonomy and the challenge of achieving it. Gartner likewise predicts a rapid rise in autonomous decision-making: by 2028, at least 15% of day-to-day work decisions will be made autonomously by AI, up from essentially 0% in 2024.
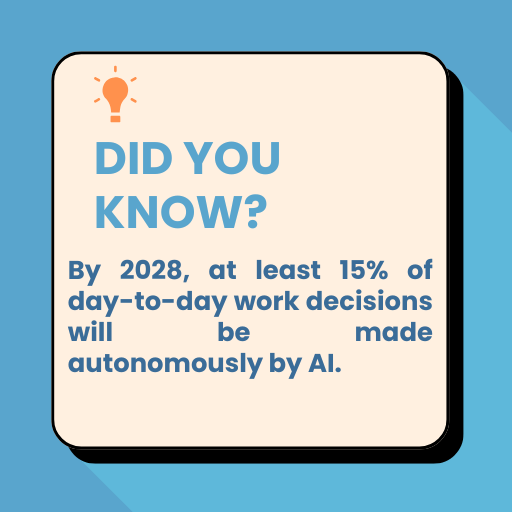
Source: Infographic by turian. Data from Gartner.
In short, advancing through the levels of AI autonomy is increasingly seen as key to competitiveness, enabling businesses to boost efficiency, improve decision quality, and operate at new scales. Leaders in manufacturing, supply chain, logistics, and beyond are especially interested, since these sectors involve myriad repetitive or complex tasks that AI can learn to handle. To better grasp how AI evolves from basic automation to full autonomy – and what it means for businesses – we can break down the journey into 5 levels of AI autonomy, drawing an analogy to the well-known levels of autonomous vehicles. Each level represents a step-change in the AI’s capabilities, intelligence, and the amount of human oversight required.
Level 1: Basic Automation
Level 1 autonomy is the starting point of the AI spectrum – essentially basic automation driven by fixed rules and scripts. At this stage, an AI or system follows predetermined instructions to execute simple, repetitive tasks, with minimal intelligence beyond what’s explicitly programmed. There is no learning or adaptation; the system cannot deviate from its rulebook. This is akin to a car’s basic cruise control or a manufacturing machine on a fixed program. In business, Level 1 includes technologies like robotic process automation (RPA) bots that perform routine data entry, invoice processing or order fulfillment steps based on set rules. For example, an RPA bot might log into an inventory system every hour and copy data to a spreadsheet – tirelessly and error-free, but completely dependent on predefined rules. Similarly, a simple robot arm on a factory line that tightens the same bolt over and over is operating at Level 1 autonomy. These systems dramatically improve consistency and throughput for high-volume repetitive tasks.
The significance of Level 1 for industries like manufacturing, wholesale, and logistics cannot be overstated. Basic automation is often the first step in digital transformation – it “bakes in” productivity gains that were previously reliant on humans and sets the stage for more intelligent automation. Companies in these sectors have aggressively adopted rule-based automation to handle structured, routine tasks at scale. According to McKinsey, as far back as 2015 about 64% of all manufacturing labor hours were technically automatable with then-current technology. This indicates enormous scope for basic automation to take over manual work. Indeed, manufacturing is among the most automated industries already (second only to food service in automation potential), thanks to widespread use of fixed robots and control systems.
The benefits at Level 1 are clear: improved speed, lower error rates, and freed-up human workers who can be redeployed to higher-value activities.
Risks at this basic level are relatively minimal – since the AI isn’t making independent judgments, errors usually stem from bad instructions or edge cases the rules didn’t cover. The main limitation is lack of flexibility: if something falls outside the scripted scenario, the automation fails or requires human intervention. For example, an RPA bot might crash if a software interface changes unexpectedly, because it lacks the intelligence to adapt. Businesses must therefore invest effort in maintaining these systems and handling exceptions. Nonetheless, Level 1 autonomy (basic automation) is now table stakes in many industries – a necessary foundation for scaling operations and reducing costs. It establishes the “automation mindset” in an organization, where teams begin to trust machines with routine work and develop processes around that. This lays the groundwork for moving up to more advanced AI levels of autonomy.
Level 2: Partial Autonomy
At Level 2, AI systems move beyond static rules into partial autonomy, incorporating some machine learning or adaptive capabilities. The AI can make limited decisions on its own within a narrow scope, but still requires human guidance or validation for most outputs. Think of this as the “driver assistance” stage of AI: the system can handle parts of a task using patterns and data, yet a human supervisor must remain in the loop. A Level 2 AI can learn from data to improve a specific function, but it operates under close oversight and predefined constraints. It is still task-specific, meaning the AI is designed to perform a narrowly defined function and cannot generalize beyond its training or scope. In practice, many modern business applications of AI sit at Level 2. For example, a predictive analytics model might forecast demand in a supply chain or flag anomalies in a manufacturing process. It uses machine learning to recognize patterns (e.g. seasonal sales trends or machine sensor readings) and provides recommendations, but a human planner still reviews and approves any final decisions. Another common case is computer-vision quality control in manufacturing: an AI camera system can inspect products on a line and sort out those that don’t match specifications. The AI is making a judgment (pass/fail) based on learning from images, yet often a human worker double-checks the rejects or tunes the system’s parameters. In wholesale and retail, partial autonomy might appear in intelligent reordering systems that suggest stock replenishments based on sales data – the AI suggests “order 100 units of Item X,” but a procurement officer gives the actual sign-off. Essentially, the AI is a “co-pilot” at this level, handling routine sub-tasks and augmenting human decision-making, but not trusted to run solo.
The value of Level 2 autonomy lies in its ability to enhance efficiency and accuracy while keeping humans in control. Even limited machine learning can yield big gains. For instance, McKinsey found that companies using AI for data screening or analysis can complete those tasks in seconds instead of hours, unlocking 10–20% productivity improvements in certain workflows. In supply chains, using AI-driven forecasting and planning (with human oversight) has helped companies respond faster to changes and reduce excess inventory. IDC reports that enterprises are ramping up such intelligent automation investments – global AI spending (covering many Level 2 use cases like analytics, vision, NLP) reached $154 billion in 2023, with manufacturing and retail among the leading spenders.
Benefits of partial autonomy include better decision support (humans get data-driven insights), improved consistency (the AI applies the same criteria every time), and the ability to handle a larger scale of operations than a manual approach could.
Risks at Level 2 involve the AI’s still-limited scope and the need for human verification. The models can be prone to errors if they encounter scenarios not represented in their training data. For example, a demand forecasting AI might err during an unprecedented market shock (as many did early in the COVID-19 pandemic) and suggest drastic mis-orders. Human experts must catch these outliers – which is why human validation remains a critical safety net in partial autonomy. There’s also the risk of over-reliance: if staff assume the AI is always right, they might miss its mistakes. Businesses mitigate these risks by treating Level 2 AI as decision support, not decision replacement, and by continuously monitoring accuracy. When implemented well, autonomy in AI at this stage becomes a trusted assistant, handling complex calculations and first-line decisions so that human workers can focus on strategic or exceptional cases. This human-AI teaming is a hallmark of Level 2 and a stepping stone to greater autonomy.
Level 3: Conditional Autonomy

Source: Freepik
Moving up the ladder, Level 3 autonomy represents a more advanced AI that can make conditional decisions and act independently within well-defined circumstances. The system can handle end-to-end execution of tasks under normal conditions without human input, but it knows its limits and will defer to humans or request intervention when it encounters complexity outside its remit. In other words, the AI self-drives but with a fallback: it’s autonomous until it isn’t sure what to do, at which point human oversight kicks in. This is analogous to “conditional automation” in self-driving cars, where the vehicle can drive itself on the highway but might ask the driver to take over in a construction zone. In business processes, Level 3 AI autonomy means significantly less human involvement day-to-day, except for handling exceptions.
Real-world examples of conditional autonomy are emerging across industries. In a smart warehouse or distribution center, for instance, autonomous mobile robots (AMRs) can navigate and move goods on their own, coordinating with each other to fulfill orders. Under typical conditions – known warehouse layout, clear paths, routine orders – they work without human operators, effectively managing internal logistics. If something unexpected occurs (like an obstacle on the route or a system ambiguity), the robot might stop and signal for human assistance or await new instructions. Another example is an AI-powered customer service chatbot that can handle a wide range of common customer inquiries using natural language processing and an FAQ knowledge base. Most users get their answers from the bot alone (demonstrating autonomy in handling those interactions), but if a question falls outside the bot’s knowledge or the customer is unsatisfied, the conversation is seamlessly escalated to a human agent. In manufacturing, we see conditional autonomy in processes like automated quality control and adjustment: an AI system might continuously monitor machine performance and tweak settings to optimize output. It operates autonomously, unless it detects a scenario it was not trained for or a potential equipment issue – then it alerts a human engineer to intervene. This level often relies on AI agents that understand context and boundaries. The AI can make certain judgment calls, constrained by predefined limits set by engineers or managers. For example, a procurement AI for supply chain might autonomously reorder raw materials when stock runs low (decision within limits), but it won’t change the supplier or negotiate prices – anything beyond its limit triggers a human review.
The benefits of Level 3 autonomy are substantial. Organizations can essentially “set and forget” the AI for routine operations, confident that it will not only execute tasks but also manage simple contingencies by itself. This greatly reduces the need for constant human oversight, allowing staff to handle more strategic work or supervise multiple processes at once. In logistics, conditional autonomous systems like warehouse robots have enabled faster throughput and 24/7 operations – leading companies like Amazon and Alibaba use armies of autonomous robots in their fulfillment centers to boost capacity and speed. According to industry surveys, such autonomy is becoming mainstream – 35% of supply chain executives expect their supply chains to be mostly autonomous by 2030, with that figure rising to ~62% by 2035 when including those targeting a bit later. This reflects confidence that AI can handle conditional decision-making in supply chains (e.g. automated planning, routing, and inventory management that only seldom need human judgment).
However, risks and challenges persist at Level 3. One key issue is ensuring the AI knows when to stop and ask for help. If the handoff between autonomous mode and human control is not well-designed, the system could either fail silently or overstep its competence. In autonomous driving, this is a known safety issue, and similarly in business processes an AI might make a poor decision if it misjudges a novel situation. Therefore, defining clear “guardrails” and fallback mechanisms is critical. Gartner emphasizes that effectively managing the risks of AI agents acting autonomously requires strict guardrails and oversight tools. Data quality is another concern – since the AI is making more independent choices, bad data or edge cases can lead to faulty decisions without immediate human correction. Enterprises must invest in robust AI governance at this stage: monitoring decisions, auditing outcomes, and maintaining transparency so that when humans do intervene, they understand what the AI is doing. Trust is also a factor; employees and managers need confidence that the AI will both perform well and call for help when needed, which means thorough testing and phased rollouts are important. When done right, Level 3 autonomy can dramatically improve operational continuity (things keep running through the night or through surges in activity) and scalability, with humans only addressing exceptions rather than micromanaging every task. It represents a balance where AI handles the known-knowns, and humans handle the unknowns.
Level 4: High Autonomy
Level 4 autonomy is where AI systems become highly autonomous, capable of making independent decisions in complex scenarios with only minimal human oversight. At this stage, the AI can handle most situations on its own; human intervention is rare and typically only needed for high-level supervision or extraordinary circumstances. The system is trusted to operate essentially on its own, though within a specific domain or environment defined by its design. While highly autonomous, Level 4 systems remain confined to the environments and task domains they were trained and designed for. In terms of our vehicle analogy, this is like a true self-driving car that can drive itself in nearly all conditions within a geofenced area – a safety driver might not be needed except as a backup. For business, Level 4 means the AI is running entire workflows or making significant decisions autonomously, with humans primarily monitoring outcomes rather than step-by-step operations. This level is enabled by advanced AI technologies such as deep learning, reinforcement learning, and complex neural networks that allow the system to perceive context, adapt to changes, and even strategize to a degree.
Examples of Level 4 autonomy are emerging in cutting-edge operations. Consider a “lights-out” manufacturing plant – a facility that runs 24/7 with almost no on-site human workers. In such a plant, AI-driven machines and robots coordinate production, handle materials, perform quality checks, and adjust to routine variability on their own. Human managers may remotely monitor dashboards or intervene via control systems if something truly unexpected happens, but day-to-day production doesn’t require people on the factory floor. This is not science fiction: companies have begun to achieve it. FANUC in Japan famously has a factory that’s been operating lights-out since 2001, using fleets of robots to manufacture equipment with zero human intervention on the shop floor. In the Netherlands, a Philips electronics factory runs with 128 robots and just 9 human workers overseeing quality – a nearly fully autonomous production line for electric razors. These are real instances of Level 4 autonomy in manufacturing, where humans set the goals and parameters, and the AI-powered machines handle almost everything else. In the supply chain and logistics realm, we see high autonomy in scenarios like autonomous trucking and drone delivery pilots. For example, several companies are testing self-driving trucks on fixed routes between distribution centers. These trucks, equipped with AI, can independently drive and navigate regular traffic on highways. They still have a safety driver or remote oversight for now, but functionally they are doing the driving (equivalent to a human task) with minimal intervention – foreshadowing a Level 4 autonomous logistics network. Another domain is autonomous supply chain planning systems: here, AI platforms use deep learning to continually optimize procurement, production scheduling, and distribution. A Level 4 supply chain AI could, say, receive sales forecasts and then autonomously decide how much to produce in each factory, how to allocate inventory across warehouses, and which transportation modes to use, all while respecting constraints like cost and capacity. Human supply chain planners would only step in to tweak strategy or handle severe disruptions; the AI otherwise manages the flow. In fact, EY research indicates that new tech (like generative AI) is bringing this vision closer to reality, with a majority of supply chain leaders believing in mostly autonomous supply chains within the next decade.
The benefits of Level 4 autonomy are game-changing. Businesses can achieve massive efficiency gains and throughput that would be impossible with humans in the loop at every step. A high-autonomy operation can run continuously and react in real time to data. For instance, an AI-driven factory can ramp production up or down immediately based on demand signals, without waiting for human decisions. This responsiveness and agility lead to better resource utilization and cost savings. High autonomy also often correlates with higher precision and safety – autonomous systems don’t get tired, and they can enforce safety rules strictly. In warehouses, fully automated systems have reduced accidents by keeping humans out of dangerous material-handling tasks. From a strategic view, Level 4 autonomy enables scalability: a company can manage a far larger operation or customer base with the same human team, because AI agents are doing the heavy lifting of operational decisions. One illustration comes from the “AI lighthouses” in manufacturing (an initiative by the World Economic Forum and McKinsey) – these are factories that extensively deploy AI and automation. They report not just productivity improvements but also more flexible production that can switch product lines or personalize output with minimal incremental cost. Essentially, high autonomy AI acts as a force multiplier for the organization.
With those benefits, however, come heightened risks and considerations. At Level 4, human oversight is minimal, so any mistake the AI makes can propagate widely before a human notices. Robust testing and fail-safes are critical – the AI must be thoroughly trained to handle a wide range of scenarios. Even then, there is the challenge of the “unknown unknowns.” Complex deep learning systems can sometimes behave unexpectedly, and diagnosing or correcting an autonomous system’s behavior is non-trivial (the black box problem). This raises the stakes for AI governance, ethics, and accountability. If a Level 4 AI makes a flawed decision – say, an autonomous supply chain system allocates stock incorrectly leading to major delays – determining why it happened and who is responsible can be difficult. Businesses must establish clear accountability frameworks (often keeping a human ultimately accountable for the AI’s actions) and implement monitoring that can quickly flag anomalies. Data governance is also paramount: since the AI is largely on its own, feeding it high-quality, up-to-date data and preventing bias or drift is essential to keep decisions reliable. Another consideration is security – highly autonomous systems could be targets for cyberattacks, as malicious actors might try to manipulate an AI that controls critical operations (Gartner warns of risks like “Agentic AI-driven cyberattacks” if guardrails are not in place). Furthermore, the organizational impact is significant. Level 4 autonomy can reshape job roles and even entire business models. Employees may need reskilling to work alongside AI (e.g. maintenance, exception management, AI system design) instead of doing the operational tasks the AI now handles. Companies achieving high autonomy often face cultural adjustments: moving from a workforce of doers to a workforce of strategists and supervisors of AI. Those that manage this transition well can unlock tremendous value, while those that don’t may face internal resistance or skill gaps. In summary, Level 4 autonomy offers a leap in efficiency and capability for businesses, but it demands rigorous risk management, strong governance, and change management to implement successfully.
Level 5: Full Autonomy
At the top of the spectrum is Level 5: Full Autonomy, where AI systems operate completely independently of human intervention. A Level 5 AI can handle any task, decision, or scenario within its defined domain just as a human expert would. At this stage, the line between “AI domain” and general problem-solving may begin to blur within that field, as the AI can learn and adapt to new situations on its own. However, this level is still domain-specific and does not imply general intelligence across all tasks. There are no hand-offs to humans in daily operation; humans simply set goals or high-level direction, and the AI agent figures out the rest. This is the ideal end-state of autonomy in AI, often equated with human-level performance in a specialized area, but distinct from Artificial General Intelligence (AGI), which would generalize across domains. Using the vehicle analogy, Level 5 is a car that can drive anywhere, under any conditions, without a steering wheel at all. In business, Level 5 autonomy means an AI-driven enterprise or process that runs by itself, with humans only monitoring at the highest level or focusing on strategy and innovation.
True full autonomy in real-world business scenarios is still aspirational, but we see glimpses of it in highly controlled environments or pilot projects. Fully autonomous factories – sometimes called “dark factories” – aim for lights-out manufacturing end-to-end. In such a scenario, an AI system not only runs the production machinery (Level 4), but also makes higher-order decisions: scheduling production runs based on orders, adjusting plans when raw material supply is late, performing predictive maintenance on itself, and optimizing energy usage, all without human approval at each step. While even the most advanced factories today (like the Philips and FANUC examples mentioned earlier) still keep a handful of humans for oversight and maintenance, the goal is to eventually remove the need for on-site human decision-making entirely. Similarly, in supply chain management, one can envision a fully autonomous supply chain AI that monitors global demand and supply in real time, automatically sources from optimal suppliers, balances inventory across the network, and routes products to customers using a dynamic logistics network of autonomous vehicles and drones. Some leading-edge companies are experimenting with elements of this vision. For example, early-stage AI agents can already assist with simple procurement contract negotiations within predefined parameters or dynamically reroute shipments in response to disruptions like weather events. However, these applications remain constrained and typically operate under tight rules and with human oversight. In the realm of enterprise decision-making, Level 5 could involve AI at the strategic level, such as identifying market opportunities or autonomously setting dynamic pricing strategies. We already see full autonomy in tightly scoped domains like algorithmic trading, where AI systems execute split-second decisions without human intervention. Translating that level of autonomy to broader business operations is still emerging but progressing rapidly.
The potential benefits of full autonomy are revolutionary. A fully autonomous AI system can achieve a scale and scope of operations that no human team, no matter how large, could match. It can analyze vast amounts of data, respond instantly to changes, and potentially self-improve over time by learning from outcomes. While most enterprise AI systems today still require supervised updates or retraining, the goal is to develop systems that can adapt more continuously through feedback loops. For businesses, this promises unprecedented levels of efficiency, agility, and innovation. Imagine a supply chain that automatically reconfigures itself daily to minimize cost and maximize speed – or a manufacturing line that self-optimizes every hour to eliminate any waste or downtime. In theory, full autonomy could allow companies to run incredibly lean operations with near-zero manual labor for execution. Human talent can be redirected entirely to creative tasks: designing new products, devising new business strategies, and handling relationships – while the AI “takes care of business” in the background.
Achieving Level 5 autonomy could also open new business models; for example, a logistics company could operate a fleet of autonomous trucks non-stop, offering ultra-fast delivery services without the constraints of driver work hours. Moreover, fully autonomous systems could enable personalization and micro-targeting at scale – e.g., an autonomous marketing AI that tailors and executes campaigns for each individual customer, something impractical to do manually. In short, if harnessed, Level 5 AI autonomy could be a competitive superpower, letting organizations accomplish things that are currently impossible due to human resource limits.
That said, Level 5 comes with serious risks and responsibilities. Handing complete control to AI raises profound issues of trust, ethics, and governance. At this level, businesses must have absolute confidence in the AI’s reliability because there is no safety net. Any flaw in the system’s logic, any bias in its training, or any unforeseen scenario could lead to decisions that are harmful – whether financially (e.g., a bad supply chain decision that loses millions) or even in terms of safety (e.g., a factory accident if the AI overlooks a hazard). Oversight doesn’t disappear at Level 5; it transforms. Instead of supervising tasks, humans must supervise the AI’s principles and performance – setting up strong governance frameworks, continuous auditing of AI decisions, and perhaps external regulatory oversight. The ethical dimension is also critical: a fully autonomous AI might face choices that have moral implications (like how to prioritize orders during a shortage, or how to allocate healthcare resources, etc.). Ensuring the AI’s decisions align with human values and corporate ethics code is non-negotiable, which is why concepts like AI ethics boards and rigorous AI testing protocols are gaining traction as autonomy increases. There’s also the societal impact to consider. Full autonomy in many industries could displace large segments of the workforce; while it also creates new roles and opportunities, managing the transition (retraining workers, redefining jobs) is an enormous challenge for leaders and policymakers. Many organizations approach Level 5 cautiously, often keeping a “human in the loop” for critical decisions even if the AI could do it, just to maintain accountability and trust. In fact, current best practice in AI deployment is augmented intelligence – using AI to support humans – rather than completely replacing human judgment in all cases. Level 5 autonomy likely will roll out in contained domains (like warehouses, or specific decision verticals) long before it’s ubiquitous across whole enterprises, allowing time to build trust and safety measures. Companies at the frontier of AI, such as those in advanced manufacturing or tech, are incrementally pushing toward full autonomy but often simulate and test extensively before removing human oversight. As Gartner notes, “agentic AI” (autonomous agents) are nascent but maturing quickly, and organizations should prepare by understanding the tech and managing the risks in advance.
In summary, Level 5 full autonomy is the pinnacle where AI operates with complete self-reliance, offering transformational benefits in efficiency and capability along with high stakes in risk. It’s a vision of AI-driven business that is on the horizon, with pilots and pockets of success already demonstrating its feasibility. Companies that can safely attain Level 5 in relevant areas will leap ahead of competition, but doing so requires mastering all the prior levels of AI and instituting world-class AI governance. Most organizations will treat Level 5 as a journey of continuous learning – an ultimate goal to work towards as both technology and trust in AI evolve.
Benefits and Risks Across the Autonomy in AI Spectrum
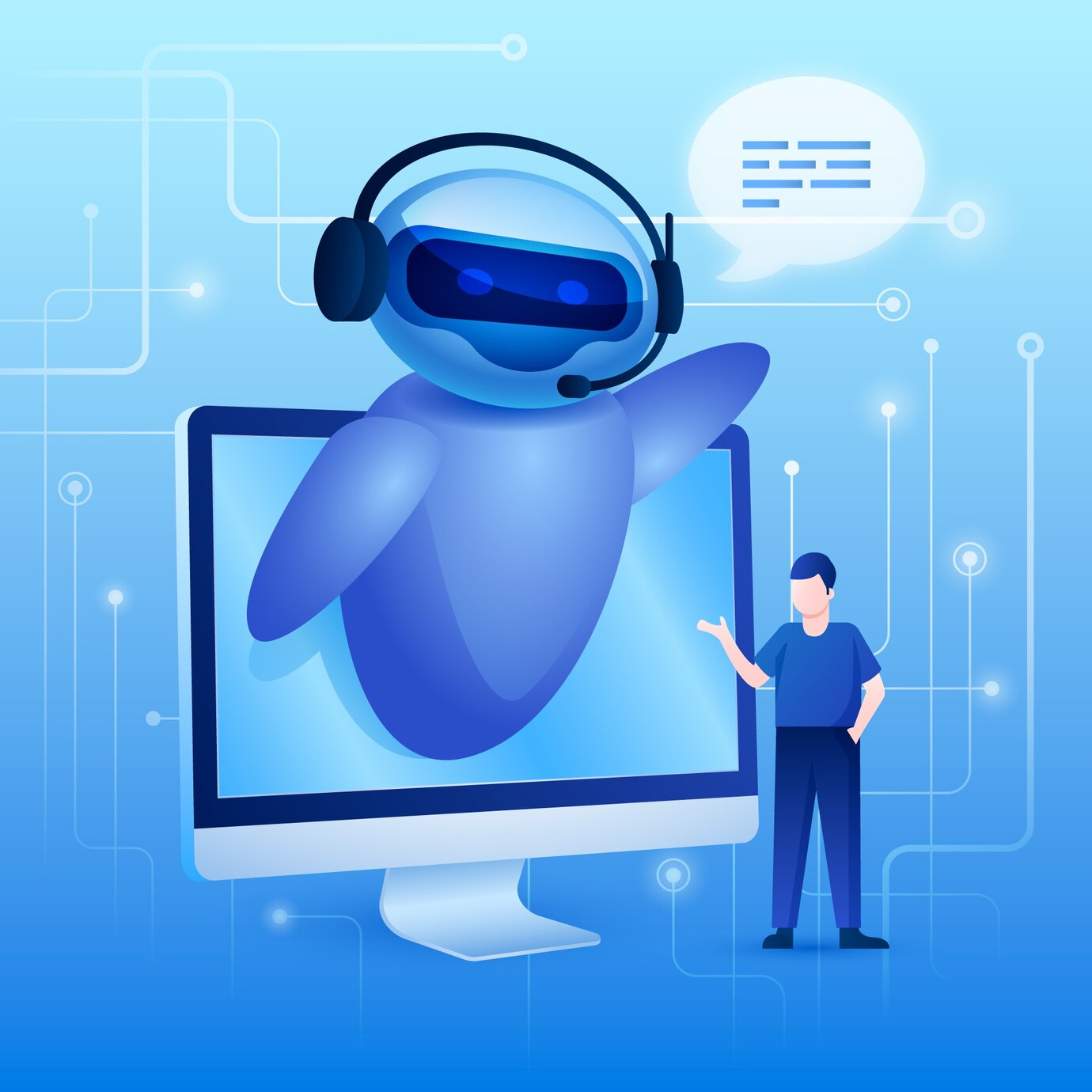
Source: Freepik
The progression from basic automation to full AI autonomy brings a trade-off between greater benefits and greater risks. It’s useful to summarize how these evolve across the five AI levels:
Level 1 (Basic Automation)
Benefits: Fast, error-free execution of simple repetitive tasks, cost savings on labor, and consistency. Frees humans from drudge work.
Risks: Very low; primarily the rigidity of rule-based systems (lack of adaptability) and failure when encountering exceptions. Managing these systems is straightforward but they can’t handle change or complexity.
Level 2 (Partial Autonomy)
Benefits: Improved decision-making support through data-driven insights (AI handles analysis), higher accuracy than manual work, and some adaptive learning leads to better outcomes over time. Humans remain in control, so quality and ethical oversight are strong.
Risks: Model errors or biases can slip through if humans over-rely on AI recommendations. Still limited in scope – the AI might give a wrong suggestion outside its training, requiring vigilant human review. Integration of these ML systems with workflows can be challenging, and poor implementation might yield little benefit.
Level 3 (Conditional Autonomy)
Benefits: Significant efficiency gains as AI autonomously handles routine cases end-to-end. Human workload drops, focusing attention only on exceptions. Operations can run longer hours with AI at the helm, increasing throughput. Faster response to known scenarios (AI doesn’t need to “ask permission” for routine decisions).
Risks: If conditions deviate from what the AI expects, errors can occur – the handoff problem if the AI doesn’t correctly alert humans. Requires well-defined limits and real-time monitoring. Governance becomes trickier: you must ensure the AI’s decisions in its autonomous zone are correct and auditable. Also, change management is needed as staff adjust to a reduced direct role (some resistance may arise if people feel out of the loop).
Level 4 (High Autonomy)
Benefits: Dramatic improvements in productivity and scalability. AI can optimize processes in ways humans might not spot, and do so continuously. Labor costs drop for operational roles, and processes can often be performed faster and at larger scale. Businesses can achieve near 24/7 continuous operation with minimal downtime. In manufacturing and supply chain, this can translate to higher output, lower per-unit costs, and increased agility in meeting demand.
Risks: Much higher dependence on AI – a single point of failure if the AI malfunctions or is compromised. Quality of outcomes is heavily tied to the quality of the AI’s learning; any flaw can have large repercussions. Harder to intervene or correct course in real time, since the AI is mostly self-governing. Organizations must invest in strict guardrails, fail-safe mechanisms, and backup plans. Regulatory and compliance issues also grow – for instance, if an AI is making decisions that affect compliance (like quality control), regulators may demand proof that the AI meets standards. At this level, ethical considerations (like fairness, transparency) become front and center because the AI’s decisions directly affect stakeholders and there’s little human mediation.
Level 5 (Full Autonomy)
Benefits: Quantum leap in efficiency and capability. AI-driven operations can achieve outcomes impossible for human-driven ones, from ultra-lean production to real-time personalization at scale. Human capital can be entirely redirected to innovation, strategy, and creative endeavors, potentially unlocking new growth. Businesses might reach unprecedented levels of speed and responsiveness, adapting instantly to market changes through AI.
Risks: Maximum risk if things go wrong – with no human in the loop, errors or bad decisions can compound quickly. Trust and reputation risks are huge: stakeholders must trust an autonomous system’s outcomes. If a fully autonomous supply chain AI makes a poor ethical choice (e.g., favoring profit over safety), the blame falls on the company. There’s also the loss of human expertise to consider: if people no longer perform a function at all, their ability to step in during an AI failure may atrophy. Companies achieving Level 5 must have contingency plans (can humans take over if needed?), continuous AI audits, and likely adhere to emerging AI regulations on transparency and accountability. Security is paramount too – a Level 5 system could be a target for hackers since controlling it could mean controlling an entire operation. Finally, the societal risk of job displacement peaks here, so businesses reaching full autonomy have a responsibility to manage the workforce transition responsibly (through retraining, new job creation, etc.).
Across all levels, a common theme is that higher autonomy amplifies both upside and downside. It’s not an all-or-nothing proposition – many organizations will determine an optimal level of AI autonomy for each process. For example, a company might push manufacturing to Level 4 autonomy for maximum output, while keeping customer service at Level 3 to maintain a human touch for complex inquiries. Hybrid “human+AI” approaches will persist, as complete autonomy isn’t always the goal or the best solution (sometimes augmented intelligence yields the best results).
One crucial point for decision-makers is that climbing the autonomy ladder should be done when the value outweighs the risk. This often means ensuring proper controls and governance mechanisms are in place at each stage before moving to the next. Implementing AI responsibly involves addressing key risks (data quality, model bias, output monitoring) in parallel with scaling up autonomy. Those companies that strike this balance effectively can enjoy the benefits at each level – from cost reductions at basic automation to strategic agility at full autonomy – without undue exposure.
Conclusion: AI Autonomy as a Catalyst for Business Efficiency and Scale
The evolution through the levels of AI autonomy – from basic automation to partial, conditional, high, and ultimately full autonomy – illustrates how AI can progressively take on more responsibility in business operations. Each step change in autonomy unlocks new efficiencies and decision-making capabilities: at first AI simply speeds up routine tasks, then it begins to learn and provide insights, later it handles scenarios independently, and eventually it may run entire functions on its own. For CXOs, Chief Sales Officers, Chief Procurement Officers, and other leaders, understanding these AI levels is crucial for setting a digital strategy. It provides a roadmap for how to leverage AI: for example, a Chief Supply Chain Officer might start by automating order processing (Level 1), introduce machine learning for demand forecasting (Level 2), then allow the AI to automatically manage inventory within set thresholds (Level 3). Over time, perhaps automated guided vehicles and intelligent scheduling systems lead to an almost self-orchestrated warehouse (Level 4). At the pinnacle, one could envision an autonomous enterprise where many daily decisions – from procurement to production to logistics – are handled by AI agents cooperating with minimal oversight (Level 5). Achieving this can result in a business that is far more efficient, agile, and scalable than its competitors.
We’ve also seen through real examples and studies that autonomy in AI is not just theoretical. Companies are already reaping benefits: higher production output, faster supply chain reactions, reduced errors, and improved customer service response times. Consulting firm analyses underscore these gains – McKinsey estimates trillions in potential productivity from AI over the coming years, and industry surveys show leaders expect mostly autonomous operations in the near future. Embracing AI autonomy, however, must be done thoughtfully. The journey is as much about people and process as about technology. Leadership needs to foster a culture that trusts data-driven AI decisions, while also maintaining the governance to monitor those decisions. Early wins at lower autonomy levels can build confidence and fund investment into more advanced AI. At the same time, addressing risks (security, ethics, reliability) early on lays a stable foundation for scaling AI. Businesses that successfully navigate to higher autonomy often follow a phased approach: pilot, learn, implement guardrails, then expand. The result is an organization where humans and AI collaborate seamlessly – AI taking care of the heavy lifting and granular decisions, humans focusing on strategy, innovation, and handling the exceptions and governance the AI cannot.
In conclusion, advancing through the levels of autonomy in AI can dramatically enhance business efficiency, decision-making quality, and operational scalability. Companies that harness even mid-level autonomy will find they can do more with less: supply chains become more responsive, manufacturing lines more productive, and services more personalized. Higher autonomy can also uncover new insights and strategies that humans alone might miss, by analyzing data and scenarios at superhuman speed. Ultimately, the goal is not autonomy for its own sake, but better business outcomes – AI autonomy is a means to achieve faster growth, higher profitability, and greater resilience in a dynamic market. As you consider applying these concepts in your own organization, it’s wise to seek expert guidance. turian’s AI automation solutions are designed to help businesses progress along this autonomy journey safely and effectively – from identifying the best processes to automate at Level 1, to implementing intelligent AI agents at Levels 3 and 4, all the way to exploring advanced autonomous systems. With the right partner and strategy, you can unlock each level’s benefits while managing the risks, positioning your company at the forefront of innovation. Embracing autonomy in AI is becoming a strategic imperative, and with careful execution, it can be the catalyst that propels your business into the future of efficiency and intelligent operations.
{{cta-block-blog}}
Say hi to your
AI Assistant!

Lernen Sie Ihren KI-Assistenten kennen!
.avif)
FAQ
Levels of AI autonomy refer to categories that describe how independently an AI system can perform tasks relative to human involvement. They range from simple rule-based automation, where the AI strictly follows predefined instructions, to advanced autonomous intelligence that can make decisions and adapt without human guidance. This framework is significant in automation because it helps organizations understand an AI system’s capabilities and limitations, ensuring the right balance between machine efficiency and necessary human oversight. For example, it’s akin to self-driving car classifications where a low level means a human is in full control and the highest level implies the car can drive itself entirely.
As AI technology matures, it progresses through these autonomy levels, becoming more sophisticated at each stage. Early-stage AI handles specific tasks with fixed rules and requires people to monitor and intervene regularly. As AI systems incorporate machine learning and more data, they begin recognizing patterns and can make limited decisions on their own, deferring to humans only when encountering unfamiliar scenarios. At the highest autonomy level, an AI system operates fully independently – it continuously learns from outcomes, adjusts its actions, and manages complex workflows without any human input. This progression illustrates how AI evolves from being a simple helper automating routine tasks to functioning as an autonomous agent that can drive processes end-to-end.
There are multiple models for defining AI autonomy, but many experts reference a framework of five distinct levels. This five-level model (similar to frameworks used for autonomous vehicles and enterprise systems) outlines a spectrum from basic automation at Level 1 to full autonomy at Level 5. Some contexts, such as self-driving cars, include a Level 0 (no automation), making it a six-level scale with no autonomy at the bottom and complete autonomy at the top. In business AI, the focus is usually on levels where AI performs meaningful tasks. Each level is defined by how much the AI can do on its own: higher levels mean the AI requires less human oversight and can handle more complex situations independently.
The distinctions between these levels lie in the degree of decision-making and learning the AI can handle, which in turn impacts automation in businesses. At Level 1 (Basic Automation), AI performs simple, repetitive tasks under strict human supervision. By Level 3 (Conditional Autonomy), the AI can make routine decisions – for example, a system might resolve standard customer requests but pass unusual or high-stakes cases to a person. At the top, Level 5 (Full Autonomy), the AI is fully autonomous, managing processes end-to-end without human input and even improving itself over time. For example, at the basic level humans still guide most steps, whereas by the full-autonomy level human involvement becomes optional. Understanding these levels helps businesses plan their automation strategy: lower levels automate routine work but still involve human checks, while higher levels enable intelligent automation that can significantly streamline operations. Solutions like turian’s AI assistants are built to achieve the higher end of this spectrum, meaning they can take on entire tasks (such as processing orders or compliance checks) with minimal human intervention once deployed.
Different AI autonomy levels directly shape how automation is applied in business operations. At lower levels of autonomy, AI tools act as assistants that automate repetitive tasks but still rely on humans for oversight and final decisions. This means businesses can speed up processes like data entry, basic analyses, or customer inquiry handling – saving time and reducing errors – while employees remain involved to handle exceptions or complex scenarios that the AI isn’t equipped to resolve. As the AI reaches mid-level autonomy, it can take on more decision-making: for instance, a system might handle the majority of routine transactions or customer requests on its own and only alert human managers when something unusual occurs, thereby improving efficiency and consistency in daily operations. In each case, the level of autonomy determines how much of the workflow the AI manages versus what still requires a human touch.
Higher levels of AI autonomy can transform business processes by enabling end-to-end automation of complex workflows. When an AI system achieves near-full autonomy, it can oversee entire processes – such as supply chain optimization, order processing, or compliance monitoring – with little to no human input. For example, turian’s advanced AI assistants illustrate this impact by autonomously handling tasks like processing orders or managing procurement steps, only involving people for strategic approvals or unforeseen issues. At intermediate autonomy, the same AI might operate in a “co-pilot” mode: it addresses standard situations independently and defers to employees for exceptions, demonstrating flexible support across various levels of autonomy in the workplace. By leveraging AI at the appropriate autonomy level, companies can automate routine work, augment employee capabilities, and gradually scale up to more autonomous operations – all without sacrificing control or quality.