
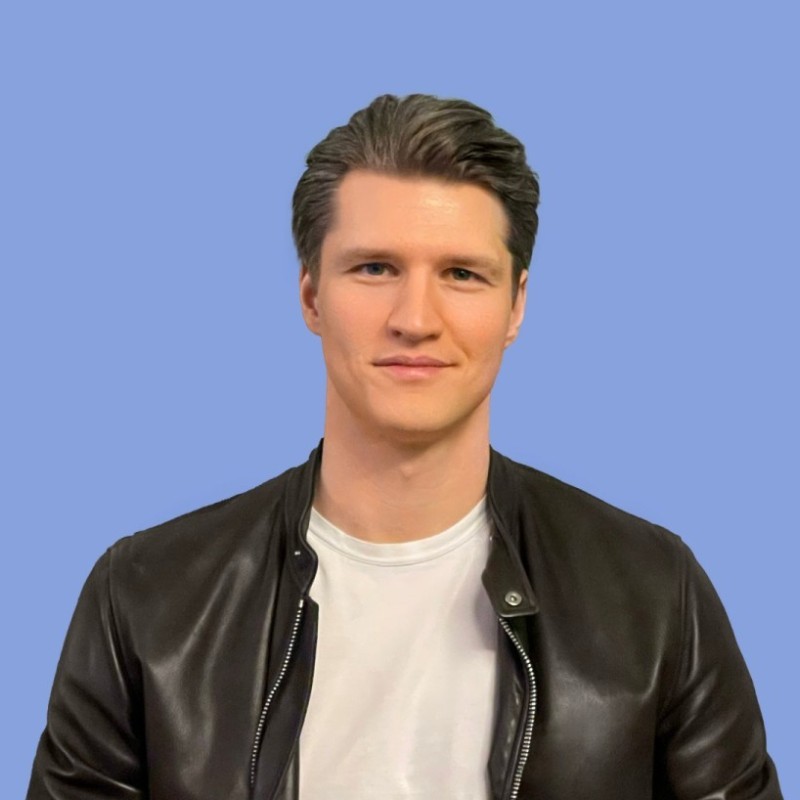
Robotic Process Automation VS AI Agents
In this age of digital transformation, businesses that want to grow, compete, and succeed must automate their processes. Automation not only speeds up operations; it frees your teams from mundane, repetitive tasks and allows them to focus on what they do their best: creatively and strategically solving problems. Two such technologies that are at the forefront of this digital transformation are Robotic Process Automation (RPA) and Artificial Intelligence (AI) agents.
But which one is the right fit for your business? The differences between RPA and AI agents still confuse many. Why? Well, because both have overlapping capabilities, and both can be used to automate business processes. However, they entirely differ in terms of their core functionalities and how they achieve automation. While RPA can be thought of as a 'robotic arm' that mimics human actions, AI agents are more like 'brains' that can think, reason, and learn on their own.
In this guide, we'll take a closer look at what RPA can do and where it falls short compared to AI agents. We'll also explore how these two technologies are different and what they bring to the table. By the end, you'll have a greater understanding of which one is better for your business needs.
RPA Functions and Capabilities: What They Can Do

Image credit: Freepik
Robotic process automation (RPA) is a form of automation technology that enables businesses to automate simple, repetitive tasks that are traditionally performed by humans. It uses software robots (also known as 'bots') to mimic human actions such as clicking, typing, and copying and pasting information across different systems and applications. These bots are programmed to follow specific rules and instructions; they only perform tasks that they have been trained to do, nothing more, nothing less. RPA can interact with the User Interface of software employees work with. For instance, an RPA bot can be “taught” a sequence of clicks in SAP or Infor that usually a human would do.
It's a "strict rule follower" that executes tasks in the same way over and over again without any improvisation or creativity. RPA is best suited for tasks that are well-defined, repeatable, and standardized in nature. Some of the most common uses of robotic process automation include:
1. Copy-and-Pasting Data
The ability of RPA to handle structured data entry is one of its key functions. It can pull highly strctured data from various sources like spreadsheets as well as third-party software, and then input that data into the designated systems or applications. By doing so, it minimizes human error and speeds up the data entry processes. RPA can also extract structured data from documents to ensure proper organization and data consistency. The rule-based nature of RPA makes it ideal for standardized data entry tasks because they are simple, repetitive, and need little to no decision-making. Importantly, RPA doesn’t understand the data it extracts and pastes, so the data input must not change over time. Otherwise, the RPA will either use wrong information or simply throw and error because it can’t find the designated data field.
2. Invoice and Payroll Processing
Invoices and payroll, in particular, can be incredibly time-consuming for employees who have to deal with field checking, copying, and calculations day in and day out. RPA simplifies these tasks by automating repetitive processes.
In the case of invoices, RPA bots are programmed to recognize and extract data from documents using Optical Character Recognition (OCR) technology, which it then transfers to the appropriate place without any human help. Similarly, for payrolls, RPA bots can be designed to effectuate multiple payments (like salaries, overtime, bonuses, etc,.) at the end of each month. These tasks are considered easy pickings- in other words, simple but time-consuming, which can be automated through RPA.
3. Pulling Data for Reports
Report generation is crucial for any business to stay on top of trends and make data-driven decisions. RPA can be a valuable tool in automating this process. It efficiently pulls data from structured sources and populates it into predefined blank fields, but that's it. RPA does not write these reports. The text body of the report remains unchanged. It just acts as a data collector and organizer. For example, you can use RPA to automatically update an Excel pie chart with quarterly data from your ERP system. It can save time and effort, but it cannot interpret the data. Human oversight is still necessary to contextualize and analyze the information generated by RPA to create meaningful reports.
4. Simple Employee Onboarding:
RPA solutions can also be utilized to automate the onboarding process for new employees. And can reduce the administrative burden on HR teams. For instance, one a new employee is hired, an RPA workflow can be designed that automatically sends the new user a series of predefined onboarding emails with instructions of how to set up their computer, how to use the printer, or how to use their employee chip card in the company canteen. The RPA system neither writes nor understands the content of those emails, it simply opens Outlook, pastes the recipient information, and then clicks send.
Limitations of RPA: Where It Falls Short
RPA is ideal for automating straightforward, rigid, processual tasks that require no creativity or decision-making, but when it comes to more complex processes that involve human-like cognitive abilities, RPA falls short. Despite its potential, RPA has its limitations that by no means make it an advanced solution for businesses that want to automate their processes. Some of these limitations include:
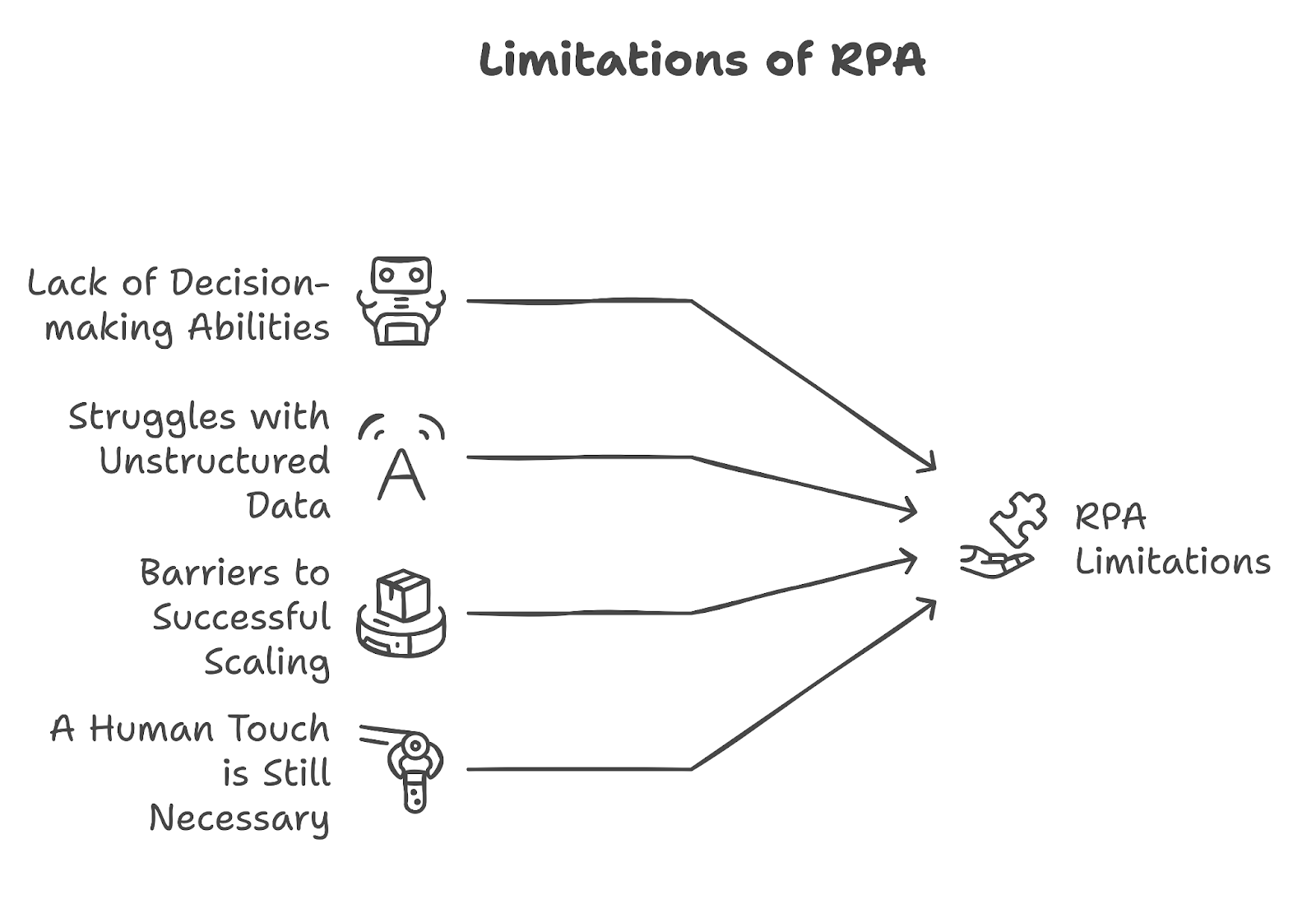
1. Lack of Decision-making Abilities
One of the main limitations of RPA is that it cannot adapt to changing circumstances or make decisions on its own. It's a brainless rule-follower. RPA sticks strictly to its instructions, just like a robot. It cannot learn, improve, or think creatively like other self-learning AI technologies. It is bound by the set of rules and instructions given to it, limiting its applicability to simple and repetitive tasks.
For instance, you can assign your RPA to extract certain fields from a document (e.g., price or customer address), but it won't be able to understand the context or recognize any errors. In fact, it won't even know which field it is extracting. For example, if the document layout changes and the customer address field is replaced by the customer's banking information, RPA won't recognize the distinction. It would mindlessly copy the banking information, mistaking it for the customer's address. This inability to learn or adapt to new situations makes RPA unsuitable for dynamic and ever-changing environments.
2. Struggles with Unstructured Data
While RPA can handle simple, rule-based tasks efficiently, it cannot deal with complex business processes that involve unstructured data. Unstructured data refers to data that does not have a predefined format or organization, such as email texts, reports, or images, making it almost impossible for classical RPA bots to extract and process. For instance, if an email or document contains a mix of text, images, and tables, RPA will extremely struggle to extract the relevant data at all.
This is because RPA cannot interpret the meaning behind the data; it can only follow a set of predefined rules. This means you need to manually adjust the rules for each specific document layout, which not only takes time but also increases the likelihood of errors and inaccuracies. It's a never-ending cycle of adjusting and re-adjusting the rules for each unique document that is not feasible for businesses that deal with a large volume of unstructured data.
3. Barriers to Successful Scaling
With RPA, scalability can become a significant barrier to success. While initially implementing RPA is quite easy, managing and governing it at scale is a different story. As your business grows, so does the complexity and volume of processes that you need to automate. This can lead to a web of interdependent bots, making it difficult to manage and maintain.
Moreover, changes to internal business processes or internal policy updates can also hinder the scalability of RPA because every little change in your workflows might require extensive re-programming of RPA functions. Additionally, many businesses still rely on outdated legacy systems that lack modern interfacing capabilities and user-friendly APIs, which can hinder the smooth integration of RPA bots. These bots are highly dependent on specific user interfaces, so any changes or updates to the UI (e.g. renaming or moving a button) can disrupt their operational flow and need time-consuming reconfiguration.
4. A Human Touch is Still Necessary
RPA is not an end-all-be-all automation solution for businesses. While it can automate simple, repetitive tasks, it cannot deal at all with complex tasks that require critical thinking, creativity, and decision-making. In fact, the success of RPA greatly depends on human input and oversight because RPA bots are only as good as the rules and instructions given to them. Without human guidance, RPA is like a car without a driver - it may work well on a straight road with little traffic, but it won't be able to navigate through unfamiliar territory or unexpected obstacles.
How RPA is Different from AI Agents
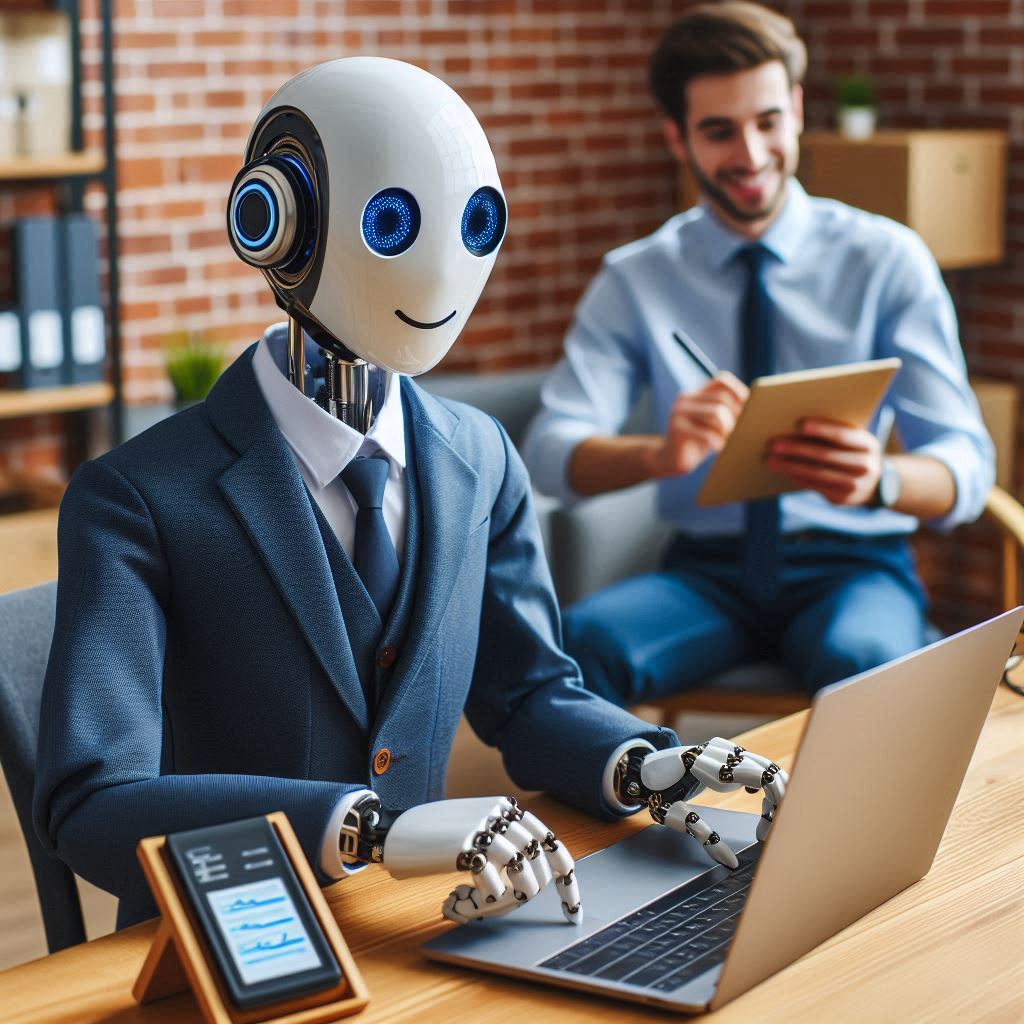
Image credit: Generated with Bing
Robotic process automation (RPA) and artificial intelligence (AI) agents are often viewed as the same thing, but in reality, they are completely different. Here's a complete breakdown of how RPA is different from AI agents:
Definition and Core Functionality
- RPA: RPA is a software technology that automates repetitive, rule-based tasks by mimicking human actions on the user interface of various systems. It does not use artificial intelligence (AI) or machine learning (ML) to do its work, but it follows a predefined set of rules and instructions given to it by humans.
- AI Agents: AI agents are a more advanced form of automation that uses artificial intelligence (AI), natural language processing (NLP), and machine learning (ML) algorithms to automate tasks. These intelligent agents can learn, adapt, and make decisions on their own with little to no human intervention.
Data Handling Capabilities
As we've said earlier, RPA is designed for structured, rule-oriented tasks. This means it can only handle data in a structured form, such as numbers or text in specific fields. It cannot understand language context or nuance, making it ineffective for data that is unstructured or requires interpretation.
On the other hand, AI agents are designed to handle any type of data, whether structured, unstructured, or semi-structured. They can work with any format of data, including emails, PDFs, Word, Excel, and even images. AI agents use NLP algorithms to understand the meaning behind data. This allows them to handle complex tasks that require an understanding of language and context.
Adaptability To New Scenarios
RPA is not a cognitive technology; it cannot learn or adapt to new scenarios. If there is a change in the environment or process, the RPA software will have to be reconfigured or reprogrammed to work in the new scenario. Even minor changes can cause a breakdown in the automation process.
AI agents, on the other hand, are well-versed in adapting to new scenarios. Advanced AI agents can adapt and continue to function without major reconfiguration when the environment changes or tasks get increasingly complex. They are constantly learning and improving through feedback loops, making them more efficient and accurate over time. For example, if an AI agent is trained to handle a specific type of invoice, it can automatically adapt to new formats and variations without any human intervention. This decision-making ability makes AI agents more resilient and efficient in dynamic business environments.
Scalability and Flexibility
When it comes to handling growth and adapting to new processes, the difference isn't solely about whether a tool uses AI or not; it's about how it is built. Both RPA and AI agents can handle high volumes of tasks. However, RPA systems might require more detailed reprogramming or reconfiguration when introducing entirely new processes or significant changes, particularly in more complex scenarios.
This can create bottlenecks and delays in scaling operations, limiting the flexibility of RPA. AI agents, by contrast, are often designed with built-in flexibility, allowing them to be trained to tackle new tasks or processes and adapt to changes more smoothly. They don't rely on pre-programmed rules and can learn from experience, making them more versatile and scalable in the long run.
How turian Can Help Streamline Your Automation Journey
If you've been looking for a more efficient, accurate, and smart automation solution that doesn't fall short in handling complex tasks like RPA, then turian is what you need. Our AI-backed agents utilize the world's most advanced LLM technology, which allows them to understand context and nuance and generate coherent responses, just like a human. Unlike RPA, which only excels in structured, rule-based tasks, our AI Assistants can handle any data, be it messy, unstructured data from various sources like emails, PDFs, Excel, Word files, and even images.
turian is not just good at automating routine and repetitive tasks like data entry, validation, and document analysis, but it can also handle more complex tasks that require natural language processing, such as drafting emails, analyzing customer feedback, or generating reports. Unlike RPA solutions that break when processes change or require constant manual adjustments, turian's AI Agents are learning and improving with new data and feedback, making them more adaptable and future-proof. turian can also flag potential errors like incorrect pricing, missing data, or wrong customer information that classic RPA solutions would miss.
This means you don't have to worry about retraining or adjusting your automation processes constantly - our AI Assistants do it for you automatically. turian comes with a user-friendly interface that allows you to monitor and control your automation processes in real time, giving you complete control and transparency. And the best part? Our AI Assistants can be seamlessly integrated into your existing ERP/CRM systems like SAP, Salesforce, and Microsoft Dynamics 365. Turian also works with popular email clients like Gmail and Outlook and can be easily customized to fit your specific business needs and industry requirements. We have incorporated a human-in-the-loop (HITL) approach to ensure that our AI agents always deliver accurate and high-quality results.
With turian, you can achieve more flexible, efficient, and scalable automation that adapts to your business's ever-changing needs. Plus, it's pre-trained and ready to use within less than two weeks. We are so confident in our technology that we offer you a POC (Proof of Concept) beforehand, where you can see firsthand how turian performs with your specific data and processes.
{{cta-block-blog}}
Say hi to your
AI Assistant!

Lernen Sie Ihren KI-Assistenten kennen!
.avif)
FAQ
The key difference between AI agents and RPA lies in how they automate processes. Robotic process automation (RPA) relies on pre-programmed rules and structured data to execute very simple, repetitive tasks like clicking, file transfers, data entry, and form filling. In contrast, AI agents (like turian) use LLMs and sophisticated algorithms to analyze unstructured data and make complex decisions in real-time.
AI capabilities go beyond RPA, as it can understand the context, learn from experience, and improve over time. AI agents can handle more complex tasks that require human-like decision-making, while RPA is limited to automating non-creative, linear, repetitive tasks that it is programmed to do; it cannot adapt or learn.
RPA cannot adapt to changes in the process automatically. It can efficiently handle very simple, repetitive tasks, but if there are any changes in the environment or process, RPA software will have to be reconfigured or reprogrammed. This means every time there is a change, the RPA process will have to be updated manually. Unlike AI agents that can learn and adapt to new situations, RPA is limited to the specific tasks it was programmed for.
Yes. AI agents can handle tasks that involve human-like decision-making. By leveraging LLMs, AI agents can understand and interpret human language, which enables them to make decisions based on context and intent. However, human intervention remains crucial in certain cases, especially when dealing with complex or sensitive matters like legal or ethical decisions. In such cases, AI agents can support human decision-making by providing data and insights, but the final judgment still lies with humans.
The choice depends on your specific business needs and operations. RPA is more suitable for very simple, rule-based automated processes. It needs clean, structured data and an unchanging environment to function effectively, which may not be feasible for businesses with complex processes or constantly changing data.
AI agents, on the other hand, can handle more complex processes and unstructured data. They continuously learn and improve from feedback, understand language context, and can handle multiple tasks simultaneously. If you don't want to be left behind in this era of digital transformation, AI agents may be the better choice for businesses looking to automate more complex tasks and processes.