

AI Vs. Generative AI: Understanding The Main Differences
Artificial Intelligence (AI) has been transforming industries around the globe. From healthcare to manufacturing, AI has redefined how businesses operate, make decisions, and deliver value to their customers. However, it's important to note that AI is not a monolithic concept; it's a broad field composed of different approaches, each serving different purposes and applications. One such approach is Generative AI- a subset of AI that has shown immense potential in reshaping industries.
So, what is generative AI? How does it differ from traditional AI? In this guide, we'll discuss the main differences between these two approaches, how they work, and what the future holds for them.
What Is Traditional AI?
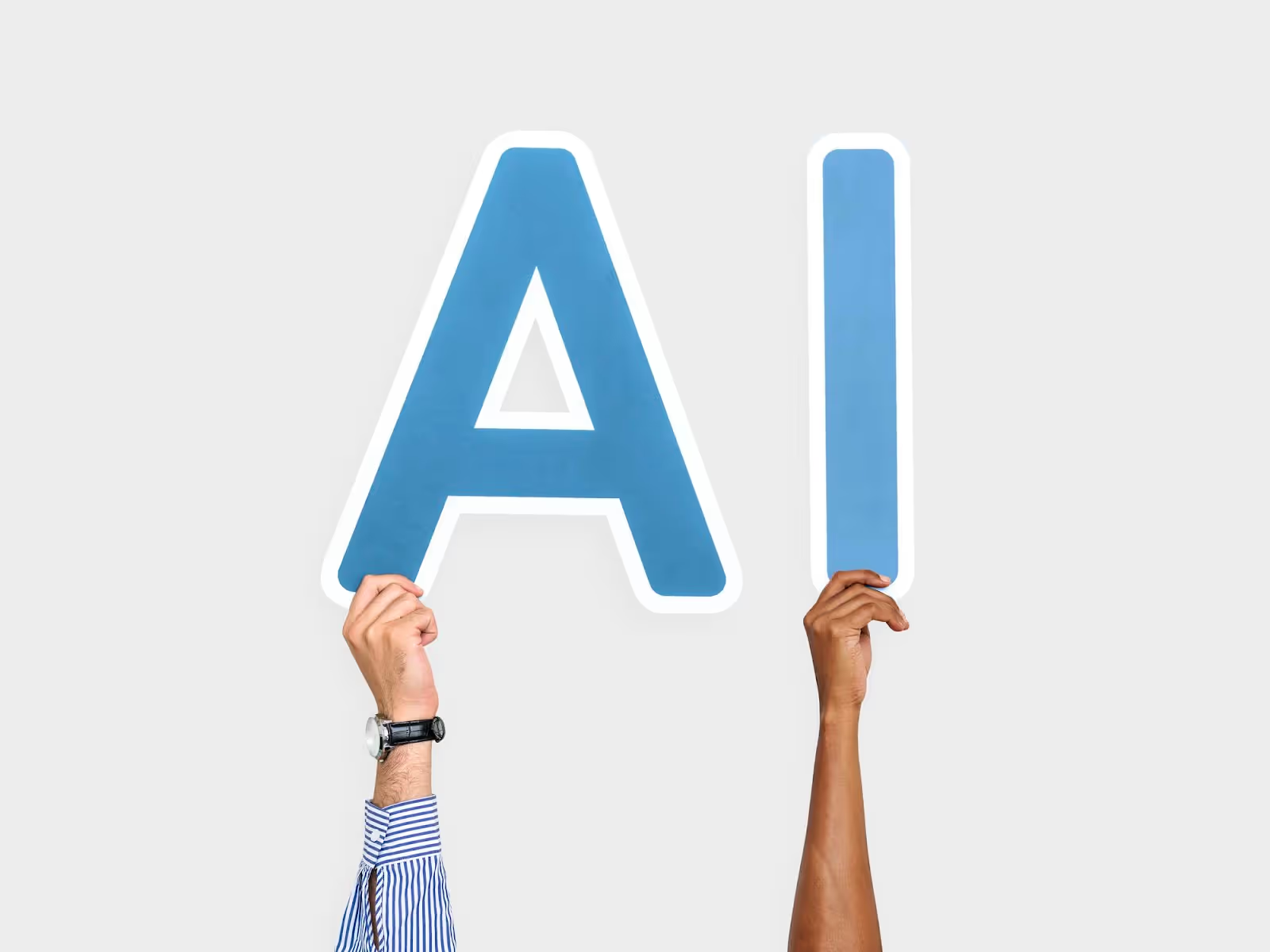
Image source: rawpixel.com
Let's start with the basics—what is traditional AI? Well, in simple terms, traditional AI is a branch of artificial intelligence that focuses on building intelligent systems that can perform structured, goal-oriented tasks. These systems are made to follow a set of predefined rules and algorithms to analyze data, make decisions or predictions, and automate tasks within their defined scope. Traditional AI systems are designed to process structured information and apply logic-based decision-making. They are programmed to operate under a given set of conditions, which makes them excellent at handling structured, rule-based decision-making across various applications.
While traditional AI can perform a range of tasks with high accuracy, it does not independently learn or adapt beyond its programmed rules. These systems can optimize their performance within their predefined logic but require human intervention to update or modify their algorithms when faced with new data or scenarios that fall outside their established parameters or rules.
Let's look at some of the main characteristics of traditional AI to understand it better.
- Programmed intelligence: Traditional AI operates based on predefined rules and algorithms created by programmers. It executes tasks within these defined constraints and does not operate beyond its programmed scope.
- Data analysis: Traditional AI focuses on analyzing large amounts of data to make decisions or predictions. It can identify patterns and trends in the data, which makes it useful for tasks like forecasting.
- Learning capabilities: Traditional AI can process data and apply predefined logic to optimize task execution. While some machine learning models improve through training, they do not generate new insights beyond their programmed scope. Any major adaptations to new or unforeseen scenarios typically require human intervention.
What Is Generative AI?

Image source: freepik
Now that we have a better understanding of traditional AI, let's move on to generative AI. What is it exactly? Well, let's define it in easy terms. Generative AI (Gen AI) is the next generation of AI that goes beyond what traditional AI can do. Unlike traditional AI, which follows predefined rules to perform tasks, generative AI is designed to create something new. Whether it's writing a story, generating images, or creating computer code, Gen AI generates outputs by identifying patterns in its training data and using probabilistic models to produce new content. So, how does it work? Well, how generative AI works is a bit different from traditional AI. Gen AI relies on advanced large language models (LLMs), transformer-based models (e.g., GPT-3 and GPT-4), or other machine learning (ML) models to learn from vast amounts of data, recognize patterns, and then use that knowledge to generate new content in a way that is similar to how humans create.
Besides data, generative AI also depends on human input, such as prompts or instructions, to create content. These prompts can be in the form of text, images, or even audio and are used to guide the AI towards the desired output. Let's look at some of the key characteristics of Gen AI:
- Generating new output: Generative AI goes beyond just analyzing data; it uses that data alongside specific prompts to craft entirely new and relevant output based on the input it receives.
- Deep learning models: Generative AI leverages deep learning techniques, including transformer-based models, GANs, and VAEs, to produce output. This is made possible through techniques like generative adversarial networks (GANs), variational autoencoders (VAEs), and transformer-based architectures like GPT-3 and GPT-4, which enable the AI to learn and generate new content based on learned patterns.
- Synthetic data: These AI models can create synthetic data that replicates real-world patterns and can be tailored to specific needs, even when actual data is unavailable. However, the quality of synthetic data depends on the model's training, architecture, and use cases. So, the better the training data, the more accurate and relevant the generated output will be.
Generative AI Vs. Traditional AI: The Main Differences
Generative AI vs traditional AI—what sets them apart? Well, let's discuss the main differences between these two approaches to get a better understanding of how they differ from each other.
Functionalities
The main difference between generative AI and traditional AI lies in how they work. While some traditional AI systems rely on predefined rules and algorithms, others incorporate machine learning and deep learning techniques to analyze data, make decisions, and automate tasks. Traditional AI systems are often designed for specific types of tasks, and they can manage a range of related tasks within their defined domain. For example, traditional AI systems can be trained to identify and classify images of dogs and cats based on features like color, shape, etc. They can also be used for more complex applications like fraud detection or risk assessment. However, if a task falls outside their defined scope, or if the underlying rules of the task change significantly, these systems require human intervention to reprogram or update their algorithms.
Generative AI systems, on the other hand, are more flexible than traditional AI, as they are not confined to specific tasks. Unlike traditional AI systems, which only follow the instructions given to them, generative AI is trained on massive volumes of data and can identify patterns, learn from them, and use that knowledge to generate new output. For example, generative AI systems trained on dog and cat images can create entirely new images that resemble dogs and cats, even if the exact images never existed before.
Applications
From healthcare to finance, traditional AI systems have a wide range of applications in various industries. They are commonly used for tasks like fraud detection, spam filtering, predicting future outcomes, classifying categories, and recommendation systems. For example, in customer service, AI-based chatbots are used to handle basic customer queries and provide support.
In contrast, generative AI systems have more unique use cases. In addition to content creation like writing, music composition, and image generation, generative AI can also be applied in various other areas. For instance, AI agents can assist with decision-making and action, achieving full automation in tasks such as updating entries in ERP systems and sending order confirmations to clients. In order management, generative AI solutions can handle a wide range of complex tasks like sales order entry, data extraction, quote generation, and order tracking. Similarly, in customer service, they can speed up response times and improve customer engagement by generating contextually relevant and personalized responses that align with your unique brand voice in real-time. Cutting-edge AI systems are not just limited to basic customer queries but can handle tasks like requesting missing information from suppliers as well.
Output Type
Traditional AI systems produce output based on predefined rules, algorithms, or learned patterns from data. These systems often rely on ML techniques, including deep learning, and their output is based on patterns learned from large datasets. While traditional AI is effective at recognizing patterns, making predictions, and performing specific tasks, its outputs are often confined to the scope of what it has been trained on. Traditional AI excels at solving defined problems, but its output is limited to familiar scenarios—it can only generate responses or actions that closely match its training data.
Generative AI, in contrast, generates novel output based on the input and data it receives. Generative AI acts as a creative assistant, generating content that appears unique and novel, even if it has never encountered the exact input before. However, it's important to remember that this "original" content is based on patterns and structures learned from its training data. Generative AI combines these learned patterns and generates novel outputs.
Model Training and Adaptability
Traditional AI systems are trained using two main techniques: supervised and unsupervised learning, though other techniques like reinforcement learning may also be used. In supervised learning, the system is trained using a labeled dataset, where both the input and output are known. In contrast, unsupervised learning involves training the system without labeled data, requiring it to detect patterns and relationships in the data without explicit instructions on what they represent. Traditional AI systems can be highly efficient at performing specific tasks, but when new data is introduced, or tasks change, retraining might be required. However, retraining is not always necessary. In some cases, models can be adapted or fine-tuned without starting from scratch.
Gen AI models are trained using various techniques, including generative adversarial networks (GANs), but other methods like variational autoencoders (VAEs) and transformer-based models are also common. GANs involve two neural networks: the generator, which creates new outputs, and the discriminator, which distinguishes real data from generated data, and provides feedback to improve the generator. This iterative process allows Gen AI systems to refine and enhance their outputs over time. While some generative AI models can adapt to new tasks and data through fine-tuning, others, such as GANs, often require more extensive retraining. The specific training and adaptation requirements depend on the model architecture and the nature of the changes.
The Future of AI

Image Source: Freepik
So, which type of AI will dominate the future? Is it traditional AI or generative AI? Well, the answer is not that simple. Both forms of AI, while having different functionalities, are not mutually exclusive. It is more likely that they will work together in the future, complementing each other's strengths and capabilities. For instance, traditional AI could analyze vast amounts of data to identify patterns and make predictions, while generative AI could use this data to create unique and personalized outputs. This collaboration could result in more advanced and efficient AI systems that can tackle complex tasks and challenges across various industries.
It's important to understand that the future of AI lies in combining the best of both worlds. Both forms of AI have their own strengths and limitations, and by combining them, we can create truly intelligent systems that can think critically, learn from experience, and generate creative outputs. So, it's not a matter of choosing one over the other, but rather recognizing their potential and using them together to unlock the full potential of AI. In short, both traditional AI and generative AI have a bright future ahead, working hand in hand to push the boundaries of what is possible with artificial intelligence.
Discover The Power of turian's Generative AI Solution
If you're looking to automate your business workflows and improve overall efficiency, turian's generative AI solution is the way to go. Our solution uses the most advanced generative AI technology, including large language models (LLMs) and deep learning algorithms, to automate repetitive tasks and streamline operations. turian's generative AI is designed to mimic human intelligence. Our solution possesses skills comparable to those of a human employee, with the ability to understand natural language, interpret complex instructions, and respond intelligently.
Whether you're processing emails, requesting missing information from suppliers, managing incoming documents like sales orders or invoices, or confirming orders to clients, turian has you covered. While machine learning models can handle rule-based, repetitive tasks, our generative AI goes beyond that. turian can understand unstructured data, such as Word files, PDFs, Excel sheets, or images, and adapt to changing inputs, making it more flexible and dynamic.
One of the best things about turian is that it requires no lengthy training processes. Our models are highly adaptive and ready to use straight out of the box, ensuring faster deployment and immediate results. With turian, you can start automating your business operations without any hassle. With its agentic AI capabilities, turian can also make decision-making processes and take actions autonomously. Our AI assistant can handle tasks end-to-end without any human intervention, from updating entries in ERP systems to sending order confirmations to clients.
By automating entire workflows, turian helps businesses drive efficiency, reduce administrative overhead, and improve customer experience. Plus, turian's enterprise Generative AI tool comes with a proprietary AI Safety Layer and customizable Business Rules, giving businesses full control over how the AI behaves. You can set specific triggers, workflows, and actions that align with your business needs, ensuring compliance and precision across all automated tasks. If you want to see how turian can streamline your business operations and boost productivity, we offer a free Proof-of-Concept so you can experience the power of our Gen-AI solution firsthand.
{{cta-block-blog}}
Say hi to your
AI Assistant!

Lernen Sie Ihren KI-Assistenten kennen!
.avif)
FAQ
Generative AI works by using deep learning models, such as transformer-based neural networks or generative adversarial networks (GANs), to analyze vast amounts of data. These models identify patterns and structures in data and use probabilistic modeling to generate outputs that appear novel while adhering to the learned structures in their training data. What makes generative AI stand out is its ability to generate context-aware content. By responding to specific user prompts, it can produce outputs that align with the given instructions, whether for writing, images, or even code. While it doesn’t create solutions in the problem-solving sense, it generates responses that align with user-defined instructions and learned data patterns. Think of it as a tool that goes beyond preset tasks, generating outputs that appear new by leveraging learned patterns. At its core, generative AI models recombine elements in ways that align with their training data, creating outputs that seem innovative while staying within the boundaries of the knowledge they’ve been trained on.
Generative AI applications in businesses are diverse and impactful. This AI can generate creative content such as text, images, and audio by identifying patterns in its training data and producing outputs that appear novel. It can also be guided by user prompts to personalize content and improve customer experiences.
Whether used for product design, marketing strategies, customer service, or operational efficiency, generative AI is transforming workflows across various departments, including sales and marketing. With Gen AI systems, businesses can gain deeper insights into user behavior, automate routine tasks, and support creative and strategic decision-making. The types of content generative AI can create are varied, from written articles to visual designs and even code, all generated in response to human language in ways that mimic expert-level execution.
Traditional AI systems rely on predefined rules, algorithms, and machine learning techniques to perform specific tasks within a fixed framework. While these systems can efficiently handle tasks they’ve been trained on, they struggle with new data or unexpected tasks without retraining or human intervention. If the task changes significantly, traditional AI requires manual updates or modifications to adapt.
In contrast, generative AI does not rely solely on explicit rules and is designed to generate new content, learn from patterns, and adapt to varying inputs. However, it is not entirely self-learning—it requires fine-tuning and reinforcement from user prompts or feedback to maintain accuracy and relevance.
Advanced Gen AI tools, like turian, come pre-trained and often require minimal customization for new or unique tasks. From predictive analytics to requesting missing information from suppliers, turian AI solutions automate a range of business processes with reduced human intervention, allowing for greater efficiency and adaptability in dynamic business environments.
Yes, turian's generative AI solution is highly customizable to meet specific business needs. Our proprietary AI Safety Layer and customizable Business Rules give businesses control over how the AI behaves and performs tasks. With turian, you can set specific triggers, workflows, and actions that align with your business processes and needs. This not only ensures compliance but also allows for increased precision and efficiency in automated tasks. Plus, our team works closely with clients to understand their unique needs and tailor the AI solution accordingly.